David Ping is the author of The Machine Learning Solutions Architect Handbook we got the chance to sit down with him and find out more about his experience of writing with Packt.
Q: What is/are your specialist tech area(s)?
David: Over my 26 years of experience working in financial services and hi-tech, I have had the opportunities to work on many different applications and technologies. I have designed and built large-scale financial services applications, created enterprise-scale cloud architecture, developed ML solutions, and implemented enterprise-scale ML platforms.
Q: How did you become an author for Packt? Tell us about your journey. What was your motivation for writing this book?
David: When I was approached to author a book on machine learning, I became motivated in writing a book to share my learning as a ML Solution Architect (SA). ML SA a relatively new discipline in the ML field, so I wanted to share my experience on what it is and what technical knowledge and skills someone will need to become an effective ML SA.
Q: What kind of research did you do, and how long did you spend researching before beginning the book?
David: I have a broad interest in ML including algorithms, learning methods, bias and fairness, and ML privacy. I am especially interested in ML research topics that make human knowledge more accessible such as ML-based knowledge graph applications. I have spent many years working on various ML related topics spanning a number of industry use cases, ML science, ML system engineering, and ML operation..
Q. What’s your take on the technologies discussed in the book? Where do you see these technologies heading in the future?
David: ML has moved beyond just modeling. In the recent years, more emphasis has been placed on ML system engineering and ML operations to speed up ML project deliveries and ML product ionization. Companies across many industries are also adopting public clouds for ML initiatives to achieve the scale, speed, and elasticity offered by public clouds. This trend will continue as more and more companies start to adopt ML into their business operations. Both open source technologies and commercial products will continue to advance rapidly to meet increasing demand in the space. ML models will become bigger and smarter to solve problems that were not possible to solve previously.
Q: Why should readers choose this book over others already on the market? How would you differentiate your book from its competition?
David: Instead of focusing a particular ML technology or a ML use case , this book looks at the various ML business, science, data, technology, operations, and governance topics through the lens of the ML solutions architecture practice. It is designed to help readers get a broad and deep view across multiple relevant knowledge and skills to practice ML solutions architecture. The book will cover benefit readers who are interested in a subset of ML topics such as industry workflows and use cases, ML governance, advanced ML engineering.
Q. What are the key takeaways you want readers to come away from the book with?
David: The ML SA function is playing an increasingly important role in the end-to-end ML lifecycle across many industries. It is one of the best times to get into the ML domain and develop a career in this area. To be successful in this domain, you will need to develop both the depth and breadth to help move ideas into paratactical ML solutions or products.
Q. How would you describe your author journey with Packt? Would you recommend Packt to aspiring authors?
David: The team at Packt has been a great partner in my journey as an author. I was provided all the necessary support to be able complete this book. I highly recommend Packt to aspiring authors who are interested in publishing technology related books.
You can find David’s book on Amazon by following this link: Please click here
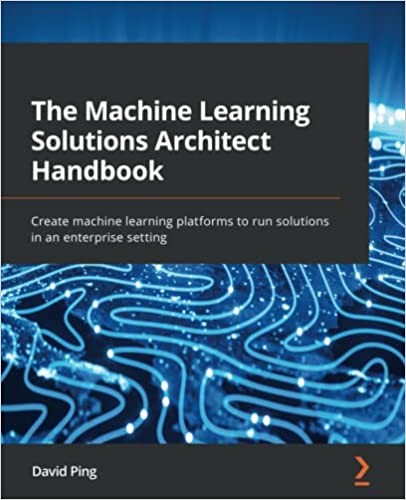